Highlighting Faculty Member Yi Feng
scarusoucla
Date published: 09/26/24
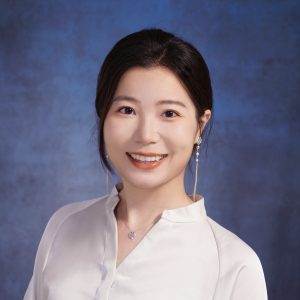
There are often gaps between the various research questions that psychology researchers seek to investigate and the availability of proper statistical tools that can effectively address them. My work is dedicated to closing these gaps by developing innovative statistical tools that can be applied to increasingly complex applied research scenarios.
As a quantitative methodologist, my work is centered on creating and advancing innovative statistical tools that can meet the needs of increasingly more complex research questions in psychology and social science. As theoretical hypotheses become more sophisticated and data structures grow more complex, applied researchers are now facing more challenges in addressing their research questions with quantitative methods. When there is a mismatch between theoretical inquiries and methodological approaches, it can lead to missed opportunities to advance scientific knowledge or, even worse, result in biased and invalid results that are misleading and hardly interpretable nor replicable. My research focuses on developing new statistical approaches and improving the existing ones, putting the right tools in the hands of applied researchers, and thus enabling them to address complex research questions that extend beyond the scope of traditional statistical methods.
My current research efforts mainly focus on developing novel analytical approaches within the structural equation modeling (SEM) framework and employing causal graphical models to understand and evaluate the common analytical models from a causal perspective. Below are a few selected examples of my published and ongoing collaborative research:
1) How to model the individual knots, floors, and ceilings as random variables in latent growth modeling?
In longitudinal/developmental studies, individual growth trajectories are sometimes bounded by a floor at the beginning of the observation period and/or a ceiling toward the end of the observation period (or vice versa), resulting in inherently nonlinear growth patterns. These type of longitudinal growth patterns can be modeled using a linear piecewise (spline) functional form, in which segments join at knots. Researchers may be particularly interested in estimating the timing of these transitions and, in some cases, estimating the levels of the floors and/or ceilings if they are unknown. Further, researchers may wish to model the knots, floors, and ceilings as predictors or distal outcomes. To address such needs, we proposed a reparameterized piecewise latent growth curve model. This reparameterized model provides a novel way to directly estimate random knots, random floors, and random ceilings within the SEM framework, offering a flexible and effective approach to modeling such nonlinear growth trajectories in longitudinal settings.
2) How to utilize post hoc planned missing data designs to deal with budget cut in longitudinal studies while maintaining the desired statistical integrity?
Unexpected budget cuts during the course of longitudinal studies have become a growing concern for developmental researchers. To address this practical challenge, we proposed the use of post hoc planned missing (PHPM) data designs as a viable solution to maintain statistical integrity despite the unexpected reduced budget. Our research developed general guidelines that can help researchers identify feasible PHPM designs within a restricted budget. PHPM designs are effective in salvaging longitudinal studies by strategically introducing missing data in a way that preserves statistical power. Additionally, we developed an R package, simPM, to assist researchers in easily implementing these designs.
3) How to model variability as a latent random variable while taking into account the measurement error?
Variability is often of key interest in various research and applied settings. Important research questions about intraindividual variability (e.g., consistency of mental status across repeated measurements) or intragroup variability (e.g., cohesiveness of attitudes among members within a team) are gaining increasing research interest. To address the research needs in modeling random variability as the key construct, we proposed a multilevel SEM-based modeling approach (MSEM-RV) where variability can be modeled as a random variable. This modeling framework is a highly flexible analytical tool that can model variability in observed measures or latent constructs, variability as the predictor or the outcome, as well as the between-subject comparison of variability across observed groups, all while taking into consideration of the measurement error. To further account for unobserved heterogeneity in random variability, we also developed a finite-mixture MSEM for modeling unobserved heterogeneity in random variability (MMSEM-RV). It specifically addresses the methodological considerations of uncovering the unobserved sub-populations that differ in intraindividual variability or intragroup variability, or sub-populations that differ in the various processes and mechanisms involving intraindividual variability or intragroup variability. It thus allows for a deeper understanding of how and why individuals or groups have differing levels of variability.
4) How to causally identify and estimate interference effects using causal graphical models and multilevel models?
Social interaction is a fundamental aspect of human societies, and in educational settings more specifically, interactions among students often result in interference or peer effects. This means that a student’s exposure to an intervention—whether in the treatment or control group—can affect their peers’ outcomes. Valid causal inference then requires an appropriate modeling of such interference effects. Ignoring peer interference not only leads to invalid and non-generalizable effect estimates but also limits the ability to design effective interventions that either leverage positive interference or mitigate harmful interference. Most of the applied research in education and other social sciences still relies on the hardly credible no-interference assumption, mostly because there lack the methodological tools that can properly take interference effects into account. Our project explores the potential of multilevel models to causally identify and estimate average interference effects. Importantly, the project integrates contextual multilevel models with causal interference graphs and linear structural causal models to effectively estimate direct individual effects as well as interference effects.
I earned my first master’s degree in applied psychology at New York University. As every quantitative methodologist would agree, “you don’t choose statistics; statistics choose you.” While being a psychology student, I was fascinated by quantitative methods and quickly fell in love with it. I was fortunately enough to find the right path for me, albeit a quite nonlinear one. I then moved to Maryland, joined a big, loving academic family of leading quantitative methodologists, and earned my second master’s and Ph.D. degree in quantitative methodology from University of Maryland, College Park. After my dissertation defense, I left the East Coast for the sunny beaches of Los Angeles along with my hamster Koala, where I joined UCLA’s quantitative psychology as an Assistant Professor in November 2023. Outside of my day job, I enjoy hiking, archery, and photography. I am also going through rigorous training in mixology (aka bartending).
Categories: Spotlight